Chapter 11
Accelerating UX research through AI: Tools, trends, and challenges
Faster insights ahead: Here’s how you can implement AI into your UX research workflows without losing the human touch.
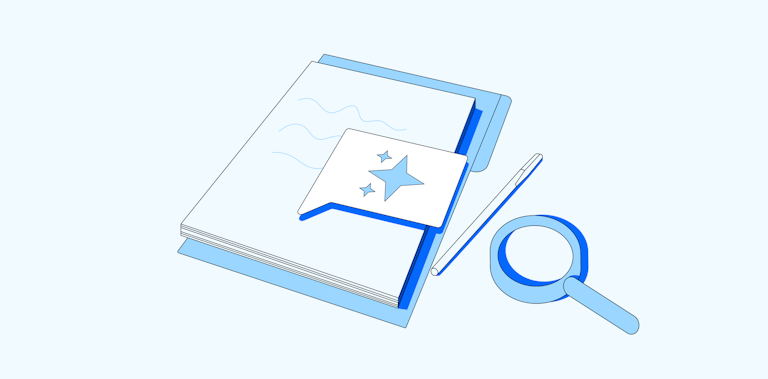
What was once a question of “Do we use AI in user research?” has now become “How do we use AI in research?”
Just a couple of years after generative tools like ChatGPT hit the market, 58% of Product teams are already using AI tools. For many, machine learning (ML) models have become a regular part of UX research workflows.
But how are teams utilizing AI as a collaborator in the UX research process? What are the challenges and considerations of adopting AI?
Read on for an exploration of how to successfully implement AI in UX research, complete with a tool for each phase of user research. We also cover the benefits, the challenges, and what you can expect from future trends.
How is AI used in UX research?
AI is used in UX research and design to streamline and automate tasks in various phases of the UX research process.
Machine learning models can:
- Write user research questions
- Find secondary research sources
- Improve data accessibility
- Analyze vast data sets
- Automate usability testing
- Co-pilot user interviews
- Transcribe interviews
- Create UX research reports
What are the benefits of using AI in UX research?
AI speeds up the UX research process by conducting otherwise manual and time-consuming tasks for UX researchers. By automating parts of the UX research process, teams can save time and resources while devoting their efforts to strategic phases of UX research.
By automating these formerly laborious tasks, we free up headspace and bandwidth to go deeper into areas where only our human brain can make magic happen. Using AI to augment research has helped us do more, faster.
Cheryl Couris
Senior Director of Design at Cisco
Share
For example, an AI model can automatically transcribe your next user interview and spot recurring codes and overarching themes, ultimately saving you time during thematic analysis. You can then use AI to turn those valuable insights into reports ready for shareholders.
The TL;DR is that AI shortens the time-to-insights and reduces much of the legwork for your team (provided you have the right tool for the job, of course).
Bear in mind that while AI can automate these tasks, and even run some of them for you independently, AI should be thought of as an aid in a UX research project, rather than an expert researcher itself.
Ultimately, AI is the co-pilot—automating the mundane tasks, speeding up manual processes, being a sounding board, and providing a second pair of hands where needed.
5 AI-powered tools for the UX research process: From ideation to impact
The market is flooded with AI research tools, many of which can help automate UX research. Some AI-enabled tools (looking at no one in particular 👀) can act as an all-in-one companion for conducting and analyzing UX research, however, you’ll likely need more than one tool to streamline your entire workflow.
That’s why we’ve searched high and low to find the best AI tools for important phases of the research process:
- ChatGPT: Discovery and ideation
- Respondent: Participant recruitment
- Maze: Conducting research
- Notion: Storing research insights
- Notably AI: Creating debriefs
1. ChatGPT: For preliminary discovery and ideation
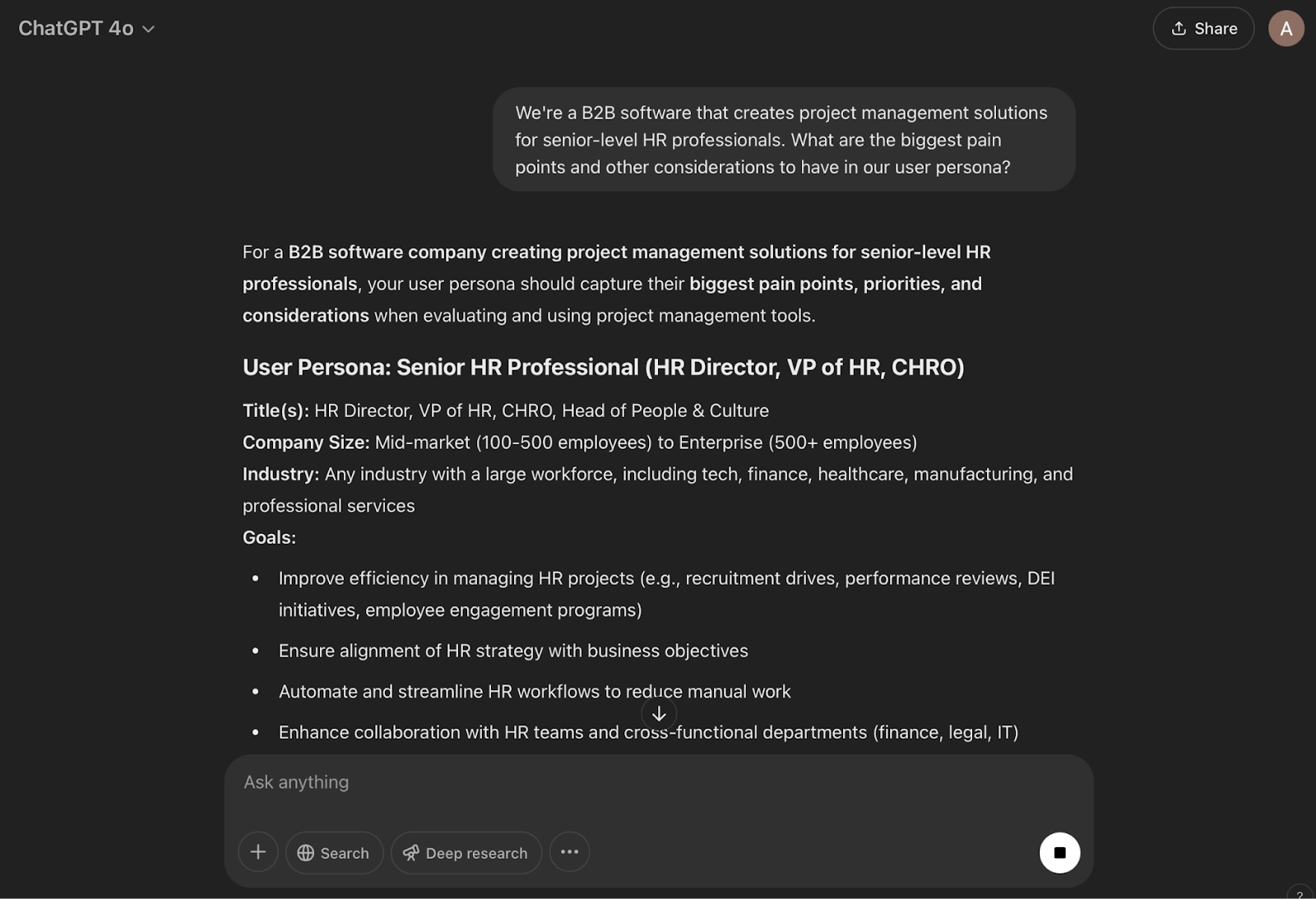
ChatGPT is a generative artificial intelligence model developed by OpenAI. It generates human-like answers to questions by analyzing a large collection of web pages and learning directly from users. The tool recently released a new Deep Research feature on its paid plans, which uses advanced reasoning to synthesize large amounts of research data and deliver thorough, reliable answers.
By feeding ChatGPT AI prompts, you can use it to conduct preliminary research and ideate for your UX research project. One example may be giving the model information on your target audience and asking it to help you create a user persona.
You can also ask it for secondary sources on market trends and even ideate UX research objectives, research hypotheses, and a rough draft UX research plan.
Pricing:
ChatGPT’s basic model is free, with a $20 Plus and $200 Pro plan for individuals, both of which include the Deep Research feature. Their Team plan for businesses is $25—it also comes with Deep Research.
✨ Pro tip
While especially helpful in the ideation phase, you can use ChatGPT at various other phases within the UX research process. For example, it can give you a rough draft of UX research questions, too!
2. Respondent: For AI-powered recruitment
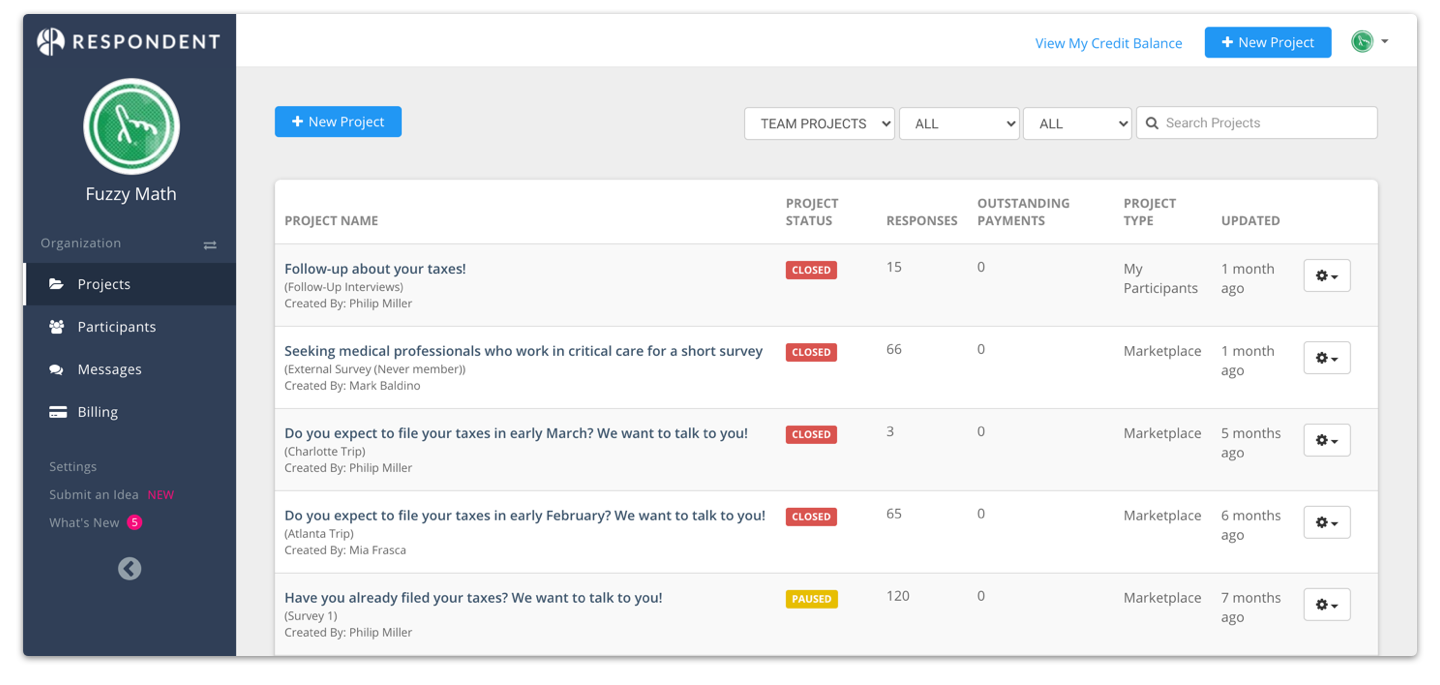
Respondent is a panel and user testing recruitment tool of over three million testers from 150 countries. However, the platform isn’t just about giving you access to potential participants. It also uses algorithms to help you sift through testers until you find the perfect candidates matching your user base.
Respondent also uses machine learning to flag ‘high-intent’ participants, effectively helping you reduce no-shows once you schedule your interview.
Pricing:
The platform has a pay-as-you-go model for $40 per session, a credit bundle model for $36 per session, and a large volume plan with custom pricing.
However, if you're already using Maze, the Maze Panel direct integration with Respondent allows you to quickly find qualified testers, directly from your research tool—at no extra cost.
3. Maze: For conducting UX research and getting insights faster
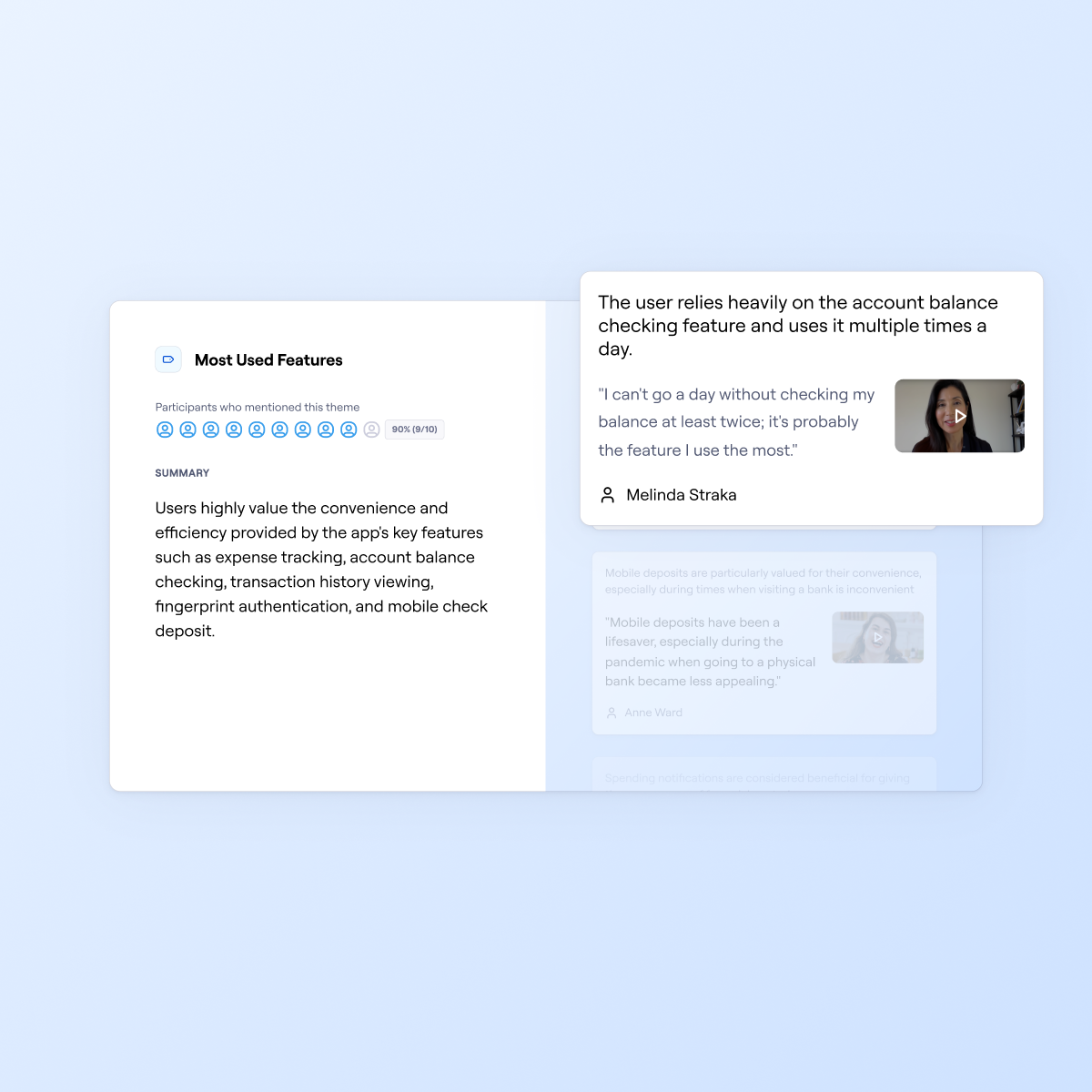
Maze is a leading user research platform with a comprehensive suite of UX research methods, many of which are AI-powered. For example, Maze’s Perfect Question feature lets you eliminate bias from your user interview questions or survey questions, which is crucial for ensuring accurate data.
When you need to dig deeper, Maze AI lets you go beyond surface-level insights by creating follow-up questions tailored to each participant's unique responses. Maze AI also automatically generates user interview transcripts after Interview Studies, and does the heavy lifting of user interview analysis, by highlighting key quotes, labeling speakers, identifying sentiment, and conducting AI thematic analysis.
Pricing:
Maze has a Free plan, a $99 Starter plan, and a custom Organization plan. Maze AI also has custom pricing.
Get started with AI-powered UX research today
Find out how Maze AI can streamline your research process from start to finish.
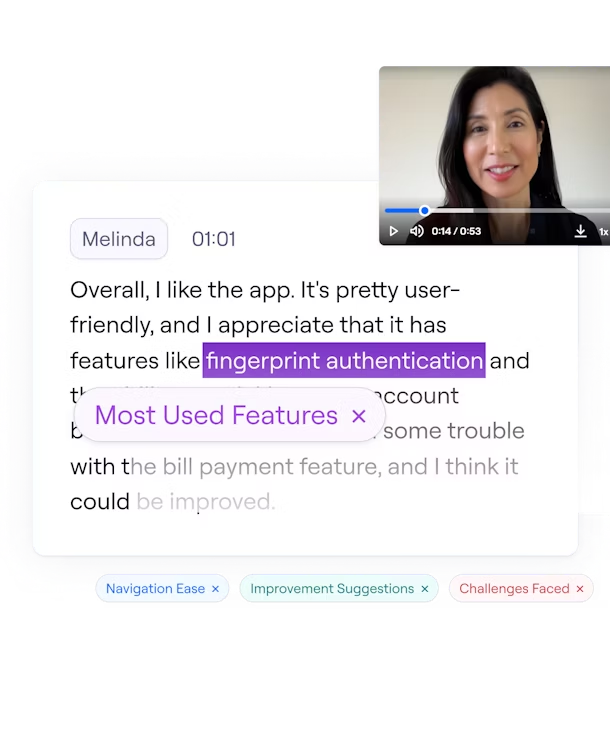
4. Notion AI: For building research and data repositories
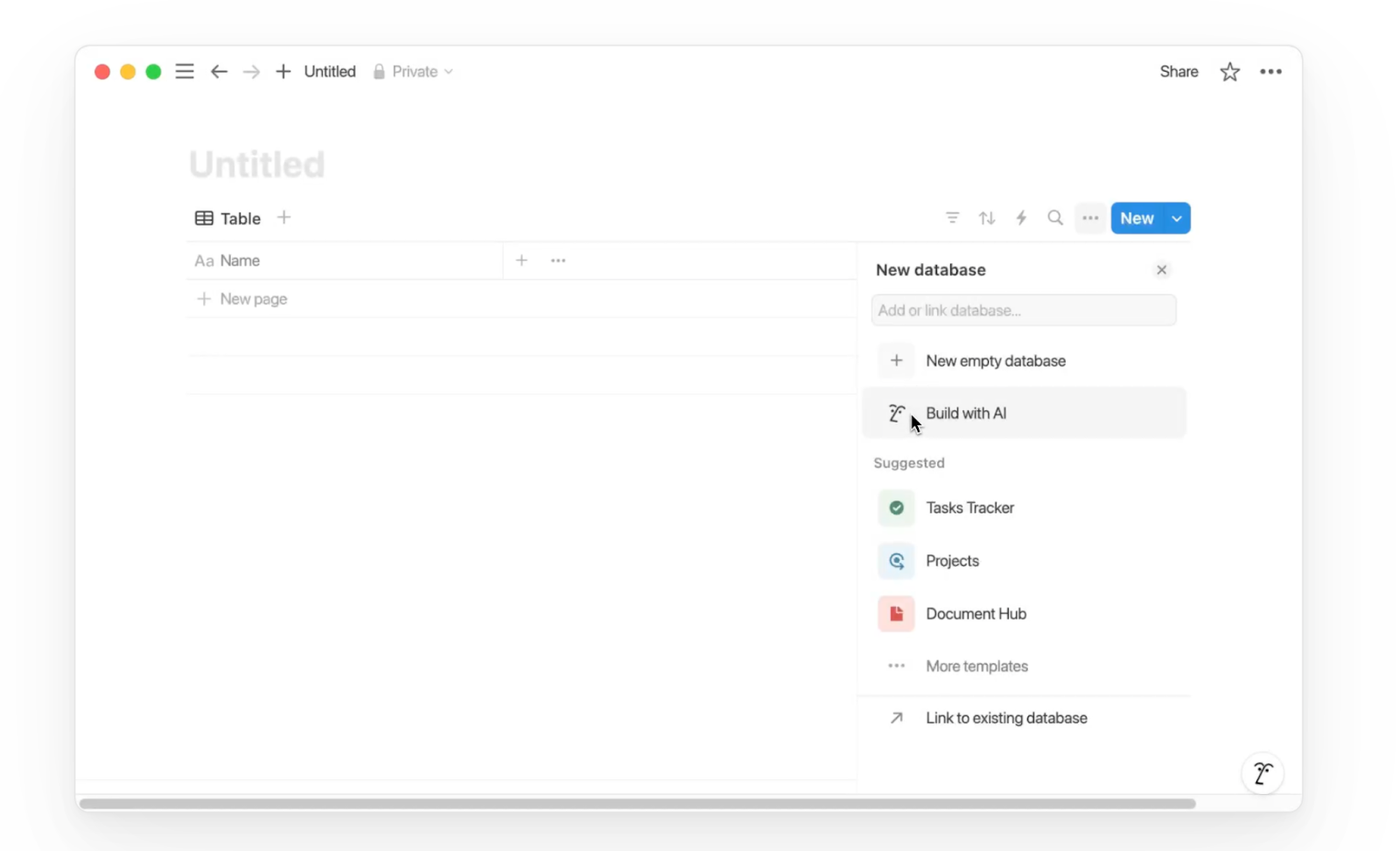
After conducting your UX research, you’ll need a secure place to store, organize, and clean your data. While dedicated repository tools exist, many UX teams fall to manually maintaining a Google Sheets or Microsoft Excel database.
With Notion AI, you can automate much of the process needed to build and set up your UX research repository—simply by describing the task to their model.
Notion AI will suggest properties, complete with generated tags and categories. Once set up, you can ask Notion to pull in results from other pages and databases, and then interlink these with future research projects. Goodbye, searching for research you swear you did a year ago, hello to intuitive insight organization.
A number of researchers are already using Notion for their research repositories, and you can even try free templates here.
Pricing:
Notion AI has a free plan, a $10 Plus plan, a $15 Business plan, and an Enterprise plan with custom pricing.
5. Notably AI: For creating insight debriefs
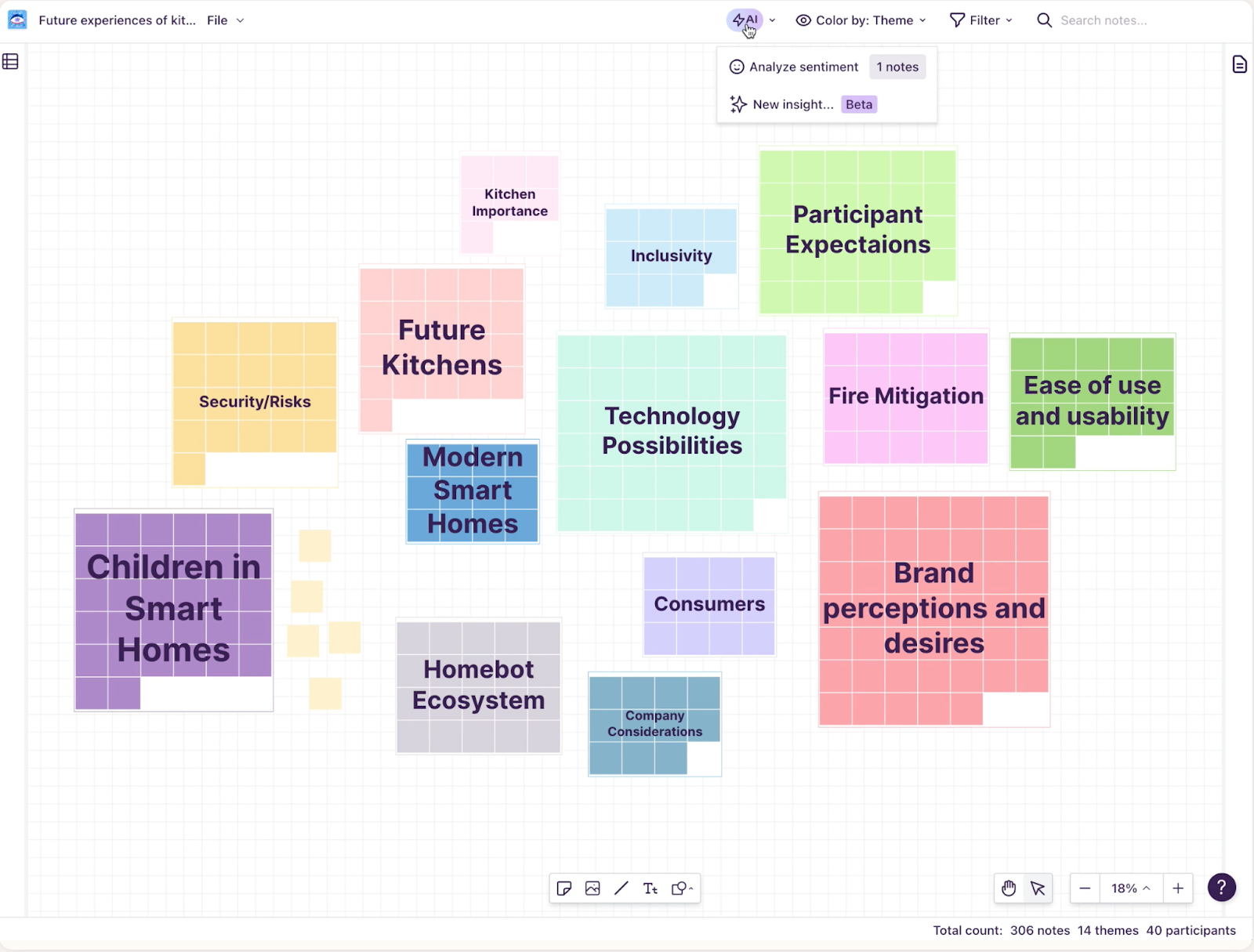
Once you’ve baked up a set of useful insights, you’ll need to divide them into digestible chunks for stakeholders. Building research reports takes time—and while AI tools like Maze can take that task off your plate—sometimes getting buy-in from stakeholders is as easy as drilling the data down into bitesized insights and key soundbites.
Notably AI makes extracting important findings easy by summarizing your qualitative data into debriefs and reports. You can use the Templates feature to ask anything about your data and get the answer quickly, while their generative image AI helps you create one-of-a-kind image content for your report.
Pricing:
Notably has a Pro plan for $50 monthly, a Teams plan for $400 monthly, and an Enterprise plan with custom pricing.
Want to keep everything in one place? Maze’s Automated Reports pull findings directly from your research study—reducing the manual steps you need to take to share findings with stakeholders.
What are the challenges of using AI in UX research?
Use AI correctly, and you have a valuable tool—one that can streamline UX research, automate repetitive tasks, and minimize the mountain of laborious admin that comes with conducting a UX research project.
But like with any other tool, notable challenges will stand in your way of mastery.
Over-reliance on AI technology
UX research is a deeply human endeavor, one that often requires immersing yourself in user feedback to better understand attitudes toward your product.
AI can reduce much of the manual work surrounding analysis, but it's precisely by conducting these tasks that many researchers form an in-depth understanding of their user base. Digging through qualitative interview data, for example, helps you come to grips with user pain points and motivations from the users’ point of view.
While you can still use AI to automate and speed up analysis, make sure to devote time to truly hearing your users’ and developing a human perspective—instead of taking results at face value.
The most important thing is to remain a critical reviewer of the AI’s outputs. If you turn off your critical thinking, then you are no longer the driver of your research and it might not meet your needs. As long as you remain critical, then I don’t think you can overrely on AI.
Peter Kildegaard
Senior Product Manager at Maze
Share
Data security for sensitive user information
It’s the responsibility of UX professionals to find tools that take strong security measures and emphasize data privacy as part of their offerings.
As such, always prioritize tools that are transparent with their data collection practices—especially ones that formally follow regulatory laws like GDPR (General Data Protection Regulation) and CCPA (California Consumer Privacy Act).
Peter also cautions against using data to train internal AI tools: “The same principles exist as with regular use of software for research purposes; you need to make sure that you own the data, and that the data is not being used against the user’s wishes.”
Potential bias in AI-powered results
Another prime concern for many UX researchers is bias and discrimination. Generative AI models are trained on large datasets, many of which contain bias and underrepresent entire demographics and marginalized groups. Not to mention, like any technology, AI is built by humans—and thus victim to our own inherent biases.
Inclusive design and UX research ethics are imperative to building positive user experiences.
This is why fact-checking is especially important when using generative AI models like ChatGPT for your ideation phase. For example, if you’re citing a study on user pain points to create a persona, it’s always best to examine its credibility, participant groups, and standards. Make a conscious, intentional effort to be inclusive in your participants, and any secondary research you’re using.
4 Best practices for AI in UX research
Although automation is a big benefit of AI, adopting ML models into your workflow isn’t an initiative you can put on autopilot. To ensure your AI adoption helps—and doesn’t hurt—your workflow, we put together this list of best practices based on our experiences at Maze.
1. Start small and scale incrementally
AI is not a replacement—it’s an augmentation. When introduced correctly, it can give you efficiency gains and save time. Choose the wrong model or replace too much too soon, and AI can end up harming your workflow and wasting your resources.
The best way to introduce AI is in small, incremental steps. Look for manual tasks that are taking up too much of your team’s time, then experiment with AI and test the results. This ensures you have a controlled environment, and you can scale more with lowered risk.
2. Comply with ethical research standards
When using AI-assisted user research, ensure you’re always collecting, analyzing, and applying data with integrity and prioritizing users’ rights. This is where consent forms, transparency, and secure storage protocols play a huge role.
AI can analyze massive sets of data, and if participants don’t fully understand how AI collects and uses that data, it’s the responsibility of UX researchers to transparently explain and clarify their intent.
There are no clear guidelines on how to use AI or when it’s acceptable to use it. I think best practices will have to evolve in the same way we’ve developed best practices in participant management, ensuring we don't use AI in an unethical way or share misleading data.
Geordie Graham
Senior Manager, User Research at Simplii Financial
Share
3. Audit your AI-generated findings for accuracy
In most cases, AI can analyze large data sets accurately, whether qualitative or quantitative. But that doesn’t mean you should take your results at face value.
Periodically check your qualitative and quantitative data by selecting a small group and analyzing it manually. Not only does this ensure accuracy, but it also lets you immerse yourself in user attitudes and helps you form a deeper understanding of their needs.
Peter from Maze emphasizes the importance of traceability when checking for accuracy:
“Your findings must have a direct connection to the source data to easily review the relevancy and correctness of the findings. You can even cross-check between models to increase validation.”
4. Combine AI-driven quantitative insights with qualitative analysis
AI-driven UX research reduces time spent on repetitive tasks—and analysis is one such task. Since models can crunch quantitative data much faster than you can, your team will have more time to gain deeper insights.
Instead of conducting purely quantitative studies like closed-ended Feedback Surveys, ask your users open-ended questions, too. You’ll end up with richer, contextual insights that not only tell you what users think but also why they think it.
Peter describes how AI often excels in pattern-matching large amounts of data for quantitative insights and pulling out examples for qualitative conviction:
The analysis in the ‘open question’ block in Maze is a great example of this, making it easy to find patterns in hundreds of responses while giving you easy access to examples.
Peter Kildegaard
Senior Product Manager at Maze
Share
The future of UX: How AI will shape UX research
As UX researchers increasingly adopt AI models into their workflows, AI tools continue to scale and innovate new capabilities that can greatly improve your research process. Just some of the future trends you can expect are:
- Predictive UX research: Instead of relying solely on historical data sets and user feedback, AI models will be able to identify patterns and predict user needs before they arise
- AI moderators for user interviews: Truly becoming the co-pilot of a UX researcher, advanced AI models will be able to conduct moderated interviews on behalf of human researchers; meaning AI can truly run the process from question creation to analysis
- Real-time UX feedback loops: As models become more advanced, they may be able to analyze user behavior in real time and immediately make recommendations for usability improvements and decision-making
- Automated persona creation: As of now, researchers need to do manual research to understand their user base and create accurate personas—soon, AI will be able to aid in the process by automatically analyzing interactions before segmenting and clustering them into highly specific groups
AI can become a really important tool in increasing and augmenting human capacity. If we push for ethical behavior and appropriate safeguards, it’ll be like the switch from using paper to using computers.
Geordie Graham
Senior Manager, User Research at Simplii Financial
Share
How Maze’s AI functionalities support UX research
No matter how you intend to adopt AI into your user research workflow, so much depends on the tool you choose for the job. We may be biased, but Maze is the perfect fit—helping you integrate AI seamlessly into your research process and reducing your time to insights.
Here’s how:
- The Perfect Question for bias-free research: Automatically eliminate grammar and spelling mistakes, cognitive biases, and leading questions from your interviews and surveys
- Dynamic Follow-Up for deeper insights: Let AI ask logical, contextual probing questions to help you get richer, deeper insights during interviews
- Interview transcripts: Understanding over 22 languages, Maze AI automatically translates and transcribes your moderated interviews—so you can focus on the answers
- Automatic research analysis: Whether you’re doing qualitative or quantitative studies, Maze AI turns crude user data into insights, from sentiment analysis to key themes and soundbites
- Shareable reports: Maze turns insights into sharable reports and summaries for time-stretched teams that need to scan on the go
And with that, you’re ready to lighten the load on your research team with AI-powered research. With these best practices and the right platform by your side, insights have never been closer.
Get started with AI-powered UX research today
Find out how Maze AI can streamline your research process from start to finish.
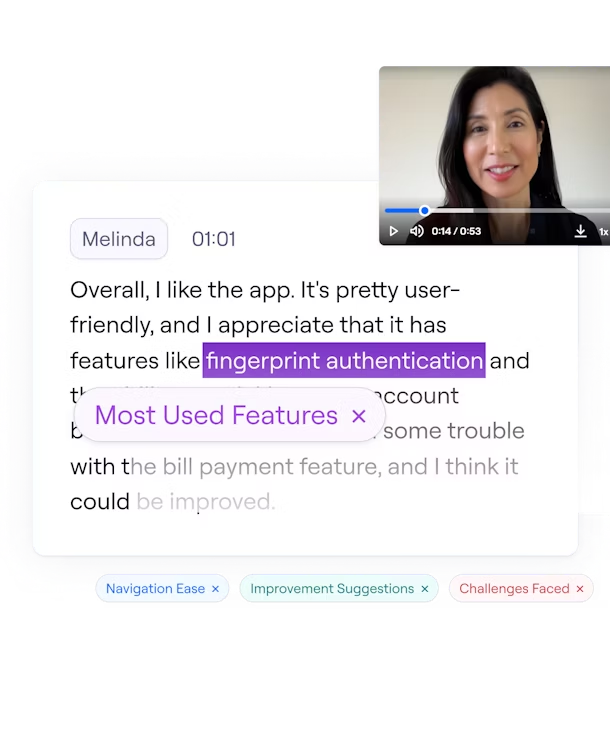
Frequently asked questions about AI in UX research
Can AI do UX research?
Can AI do UX research?
Yes… and no. AI can help users automate repetitive tasks within the UX research process, like ideating testing questions, automating analysis, and even creating reports. However, the entire workflow is still very human, as UX professionals need to strategize, design, optimize, and coordinate UX research projects to form a deep understanding of user needs and get insights.
How to use generative AI in UX research?
How to use generative AI in UX research?
Generative AI is especially useful in the early discovery and ideation phase of UX projects. Use it to aid in market research, create user personas, and even generate interview questions. Think of genAI as a “thinking partner” helping you develop ideas.
For example, you can feed ChatGPT a prompt that says: “We’re at X stage in our product design process. Based on best practices, what are some of the user research methods that could help us uncover Y?” While the model won’t necessarily give you a definitive answer, it will help you explore your options and reflect on your next steps and challenges.
Which AI tool is best for UX design?
Which AI tool is best for UX design?
How you use AI in user experience design purely depends on what you need the AI tool for. For example, Maze AI has a wide range of AI features, such as generating bias-free survey questions and follow-up questions, automatically transcribing interview sessions, analyzing data, and creating ready-made reports.